In the ever evolving world of digital marketing, Search Engine Optimization (SEO) remains a vital tool for boosting visibility and engaging users. However, as technology advances, the rise of Artificial Intelligence (AI) and Large Language Models (LLMs), such as OpenAI’s GPT series or AI-driven tools including Google’s AI Overviews, Bing’s Copilot, and Perplexity, has sparked a new chapter in content optimization. While there’s no universally agreed-upon term yet, this emerging field is often referred to as “AI Search Optimization,” “LLM Optimization,” or even “LEO” (LLM-Enhanced Optimization). It blends the traditional focus of SEO, which involves crafting content for search engines like Google, with a fresh challenge: optimizing for AI-driven systems that generate, analyze, and rank content using advanced algorithms.
This shift represents a sophisticated fusion of classic SEO tactics with the nuanced power of AI. It’s no longer just about keywords or piling up backlinks. Instead, it’s about creating semantically rich, context-aware content that aligns with how AI parses and understands text. This requires a deeper understanding of machine learning, natural language processing, and the specific ways AI tools, such as TensorFlow or BERT models, evaluate relevance and comprehensiveness. For SEO professionals, the goal is twofold: crafting content that reaches the right audience while effectively speaking the language of AI algorithms. As this integration deepens, the future of SEO depends on leveraging these advanced technologies to stay competitive, enhancing visibility and meeting the sophisticated demands of modern search systems.
Emerging Terminology – A Quick Cheat Sheet
1) AI Search Optimization (AIO)
This term is gaining traction as AI systems increasingly power search experiences. It focuses on making content discoverable and valuable to AI models that process natural language queries or generate responses.
Example use: Optimizing content for how an AI might summarize it or rank it in a conversational response.
Scenario: You run a blog about sustainable gardening, and you want your article “Top 5 Tips for Composting at Home” to be easily summarized and ranked highly by an AI responding to the query, “How can I start composting at home?”
Example Use:
Original content might be a long, narrative-style paragraph:
“Composting is a great way to reduce waste and enrich your garden soil. You can start by collecting kitchen scraps like vegetable peels and coffee grounds, then mix them with yard waste like leaves and grass clippings. Over time, this mixture breaks down into nutrient-rich compost.”Optimized content for AI:
- Clear, concise structure: “To start composting at home: 1) Collect kitchen scraps (e.g., vegetable peels, coffee grounds). 2) Add yard waste (e.g., leaves, grass clippings). 3) Mix and let decompose into nutrient-rich compost.”
- Why it works: The numbered list and straightforward phrasing make it easy for an AI to parse, summarize as “Collect scraps, add yard waste, and let it decompose,” and rank it highly for relevance in a conversational response.
2) LLM Enhanced Optimization (LEO)
This specifically refers to tailoring content so large language models (LLMs) can easily interpret, index, or prioritize it when generating answers. It’s a more technical, model-focused approach.
Example use: Structuring data so an LLM can efficiently extract key points.
Scenario: You’re creating a technical guide on “How to Install Solar Panels” for a renewable energy website, and you want a large language model (LLM) to easily interpret and extract the essential steps when answering a query like “What are the key steps to install solar panels?”
Example Use:
Original content might be a dense block of text:
“Installing solar panels involves assessing your roof’s condition to ensure it can support the panels, then figuring out how much energy you need by calculating your household usage, followed by purchasing the right equipment like panels and inverters, and finally hiring a professional or doing it yourself if you’re qualified.”Optimized content for an LLM:
- Structured format: Key Steps to Install Solar Panels: 1. Assess roof condition (ensure structural support). 2. Calculate energy needs (based on household usage). 3. Purchase equipment (solar panels, inverters). 4. Install (hire a professional or DIY if qualified).
- Why it works: The concise, bullet-pointed list with clear, actionable steps allows an LLM to quickly interpret and index the content. For example, I could extract and prioritize this as: “Assess roof, calculate energy needs, buy equipment, and install,” delivering a precise answer to the user’s query.
3) Generative Search Optimization
This targets optimization for generative AI tools that produce answers rather than just linking to websites. It’s about ensuring your content becomes the “source of truth” for AI-generated responses.
Example use: Crafting concise, authoritative answers that an AI might directly quote.
Scenario: You manage a health website and want your article “What Causes Migraines?” to be the definitive source when a generative AI tool answers the question, “What triggers migraines?” You aim for the AI to quote your content directly.
Example Use:
Original content might be a lengthy explanation:
“Migraines can be caused by a variety of factors depending on the individual. Some people experience them due to stress or changes in sleep patterns, while others might notice certain foods like chocolate or caffeine playing a role. Hormonal changes and environmental factors like bright lights can also contribute.”Optimized content for generative AI:
- Direct, authoritative statement:
“Migraines are triggered by stress, irregular sleep, foods (e.g., chocolate, caffeine), hormonal changes, and environmental factors like bright lights.”- Why it works: This concise, factual sentence provides a clear “source of truth” that a generative AI can directly quote, such as: “According to [your site], migraines are triggered by stress, irregular sleep, foods like chocolate and caffeine, hormonal changes, and bright lights.” It’s comprehensive yet succinct, making it ideal for AI-generated responses.
This optimization ensures your content stands out as a reliable, quotable answer for generative AI tools, increasing its likelihood of being used instead of just linked or paraphrased.
4) SEO for Conversational AI
A broader term encompassing optimization for voice assistants, chatbots, and LLMs, with an emphasis on natural language and question-based queries.
Example use: Writing content that aligns with how people conversationally ask questions to AI.
Scenario: You run a travel blog and want your article “Best Weekend Getaways from New York City” to rank well when someone asks a voice assistant, chatbot, or LLM, “Where should I go for a weekend trip from NYC?” You optimize for natural, conversational queries.
Example Use:
Original content might be formal and descriptive:
“There are several excellent destinations for a weekend getaway from New York City. The Hudson Valley offers scenic beauty and historic sites, while the Hamptons provide beaches and luxury, and the Catskills are known for outdoor activities like hiking.”Optimized content for conversational AI:
- Natural, question-based response:
“Looking for a weekend trip from NYC? Try the Hudson Valley for stunning scenery and history, the Hamptons for beaches and relaxation, or the Catskills for hiking and nature.”- Why it works: This mirrors how people speak to AI (“Where should I go?”) and provides a direct, conversational answer. A voice assistant or chatbot could easily respond with, “You could go to the Hudson Valley for scenery, the Hamptons for beaches, or the Catskills for hiking,” aligning perfectly with the user’s casual, question-based query.
This broader optimization ensures your content resonates with natural language searches across voice assistants, chatbots, and LLMs, making it more likely to be selected for conversational responses.
—
As we delve deeper into the implications of SEO for AI and LEO, it becomes clear that the future of SEO will increasingly rely on leveraging these advanced technologies to stay ahead in the highly competitive digital marketplace. The strategic integration of AI with SEO practices not only enhances content visibility but also aligns with the sophisticated requirements of modern search algorithms.
Why It’s Different from Traditional SEO
As of 2025, search is increasingly AI-driven. It’s possible for the past year you’ve been getting results in search, Google’s 2024 updates, for instance, integrate more generative AI into results, meaning content must appeal to both traditional crawlers and AI interpreters. LLMs don’t “crawl” sites, they rely on training data or real-time web access to find and process info. Optimizing for this means making your content AI-ready, not just search-engine-ready.
Shift to AI-Driven Search
- Traditional SEO: Historically, search engine optimization focused on making content discoverable by web crawlers (e.g., Googlebot). These crawlers systematically scan websites, index pages based on keywords, metadata, links, and structure, and rank them using algorithms like PageRank.
- AI-Driven Search (2025): Search engines like Google have increasingly incorporated generative AI into their systems. This means results aren’t just links to websites but often include AI-generated summaries, answers, or snippets (e.g., featured snippets powered by models like BERT or newer generative tools). Search is becoming more conversational and answer-focused, driven by user intent rather than just keyword matches.
Key Difference: Traditional SEO targets crawlers that prioritize technical signals (e.g., title tags, backlinks), while AI-driven search requires content to be understood and summarized by AI, appealing to natural language processing and intent interpretation.
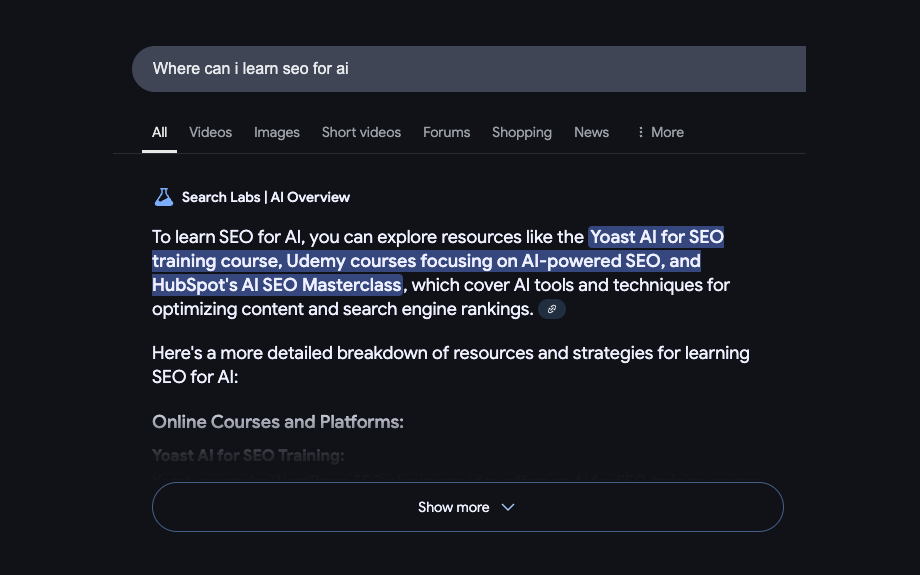
Dual Audience: Crawlers and AI Interpreters
- Traditional SEO: You optimized for crawlers by using keyword stuffing, meta tags, and site architecture to ensure a bot could index your site effectively.
- SEO for AI: Now, content must satisfy both traditional crawlers (for indexing) and AI interpreters (for comprehension). Google’s updates mean its algorithms don’t just rank pages—they use generative AI to parse content and deliver direct answers. For example, if someone searches “How do I compost at home?” Google might generate a response like “Collect scraps, mix with yard waste, and let it decompose” instead of just linking to a site.
Key Difference: You’re no longer just gaming a ranking algorithm—you’re crafting content that an AI can interpret as authoritative and useful enough to summarize or quote directly.
How LLMs Work Differently
- Traditional Crawlers: Bots like Googlebot actively scan the web, following links and indexing pages in a structured database over time.
- LLMs: Large language models don’t “crawl” in the traditional sense. Instead, they rely on pre-existing training data (a massive snapshot of the web) or, in my case, real-time web access and X posts to fetch and process information on the fly. I don’t systematically index sites—I analyze and generate responses based on what’s available and relevant to the query.
Key Difference: Traditional SEO assumes a crawler will find you eventually if your site is optimized. With LLMs, the focus shifts to making content immediately understandable and extractable, as AI might bypass traditional indexing and pull info directly from what it can access or interpret.
AI-Ready vs. Search-Engine-Ready
- Search-Engine-Ready (Traditional): This meant keyword density, fast load times, mobile-friendliness, and link-building—technical tweaks to rank higher on a results page.
- AI-Ready (New Paradigm): This means structuring content for clarity and authority so an LLM can quickly grasp key points and present them as answers. For example, a list like “Steps to Compost: 1) Collect scraps, 2) Add yard waste, 3) Decompose” is AI-ready because it’s concise and structured for extraction, unlike a long narrative paragraph.
Key Difference: Traditional SEO optimized for visibility in a list of links. AI-ready content optimizes for being the definitive answer an AI delivers, often without the user ever clicking a link.
Real-World Example:
Imagine a query: “What’s the best way to reduce stress?”
- Traditional SEO: A site might rank high with a keyword-heavy article (“stress reduction tips”) optimized with headers, backlinks, and meta descriptions. The user clicks the link and reads it.
- AI-Driven Search (2025): Google’s generative AI might respond directly: “To reduce stress, try meditation, exercise, and sleep,” pulling from an AI-ready site with a clear list: “Stress Relief: 1) Meditate, 2) Exercise, 3) Sleep well.” The user gets the answer without visiting the site, and the content had to be structured for the AI to pick it up accurately.
Why It Matters:
Optimizing for LLMs and AI-driven search requires a mindset shift. You’re not just chasing rankings—you’re ensuring your content is clear, authoritative, and digestible for an AI to process and present, whether I’m answering here or Google’s generating a result. It’s about being the “source of truth” in a world where AI doesn’t just find content—it interprets and delivers it.
Understanding the Fundamentals of LLM and SEO Integration
Definition and Importance of LLMs in SEO
Large Language Models (LLMs), such as GPT-4o, are advanced AI systems designed to understand, generate, and manipulate human language in a way that mimics human-like understanding. In the context of SEO, LLMs are pivotal because they can process and interpret vast amounts of text-based content at a scale unmanageable for humans, providing insights into keyword trends, content relevance, and user intent. This makes them invaluable tools for crafting SEO strategies that are not only responsive but also anticipatory of market trends and search behaviors.
How SEO for AI differs from traditional SEO
Optimizing for AI through LLM Optimization (LEO) significantly differs from traditional SEO practices. While traditional SEO focuses on keyword density, backlink profiles, and metadata enhancements, SEO for AI delves deeper into semantic search optimization and the creation of content that aligns with LLM interpretations of context and nuance. This requires a more sophisticated approach to content creation, where the semantic richness and contextuality are prioritized over mere keyword insertion, aiming to satisfy the advanced language comprehension capabilities of LLMs.
Impact of LLMs on Search Algorithms
LLMs have begun to reshape how search algorithms interpret and rank content. By integrating LLMs, search engines can better understand the subtleties and deeper meanings of content, leading to more accurate and contextually relevant search results. This evolution in search technology demands that SEO professionals adapt by focusing more on the quality and relevance of content rather than traditional metrics. The integration of LLMs into search algorithms means that the strategies that once prioritized keywords must now consider the holistic context and intent behind search queries, fundamentally altering the SEO landscape.
Technical SEO for LLMs: Key Considerations
Structuring Data for AI Comprehension
For SEO professionals, structuring data to be AI-friendly is crucial in ensuring that large language models (LLMs) like GPT-3 or BERT effectively understand and process the content. This involves organizing information in a clear, logical manner, often using schemas that are universally recognized by search engines and AI systems. For example, implementing structured data markup such as JSON-LD helps LLMs identify and categorize content elements more efficiently, enhancing content discoverability and relevancy in AI-driven search environments.
Keyword Optimization in an AI Context
In the realm of AI, traditional keyword optimization undergoes a significant transformation. It’s no longer just about keyword density but about context and relevance that align with the user’s intent. SEO experts must focus on semantic search optimization, where the intent behind a search query is as crucial as the keywords themselves. This means optimizing for long-tail keywords and ensuring that the content answers specific questions users might have, thereby aligning more closely with AI algorithms that prioritize user intent and contextual understanding.
Optimizing for Natural Language Processing
Natural Language Processing (NLP) capabilities of LLMs offer a unique opportunity for SEO strategies. Content must be optimized not just for keywords but for readability and natural language flow, which enhances AI’s understanding of the text. Techniques such as using natural, conversational language and including relevant synonyms and related phrases can boost SEO efforts. This approach not only aids in ranking when processed by LLMs but also improves user engagement, as the content is more relatable and easier to understand from a human perspective.
Advanced Strategies in LLM Optimization
Use of Semantic Clustering
Semantic clustering is an advanced SEO technique that involves grouping related concepts to establish topical authority in a specific domain. For SEO professionals targeting LLMs, it’s crucial to structure content by semantically related topics to facilitate the AI’s understanding and categorization processes. By implementing semantic clustering, SEO specialists can enhance the relevance of a website’s content for specific queries, which is instrumental in improving search engine rankings. This approach not only helps in organizing content logically but also boosts the visibility of clustered pages through enhanced thematic connections.
Entity Recognition and Optimization
Optimizing content for entity recognition involves identifying and enhancing significant nouns or phrases that search engines recognize as concrete concepts. This technique helps LLMs understand and index content more effectively, leading to improved performance in search results. For instance, marking up entities using Schema.org and enhancing semantic richness around these entities can significantly impact how well LLMs comprehend and relate content pieces to user queries. SEO experts must focus on refining entity salience within content to better align with LLM expectations and improve SERP positioning.
Incorporating Contextual Relevance
Ensuring contextual relevance is paramount in LLM optimization. This strategy involves aligning content with the user intent and the contextual cues within search queries. SEO professionals can leverage AI tools to analyze search trends and user behavior, thereby tailoring content that fits within the specific context of desired search queries. Contextual relevance not only improves user engagement but also signals to search engines that the content is directly responsive to user needs, thereby enhancing its authority and relevancy in related searches.
Practical Tools and Technologies for LEO
SEO Tools Adapted for AI Optimization
The adaptation of traditional SEO tools for AI optimization is pivotal in enhancing the effectiveness of LEO strategies. Tools like Moz and SEMrush have introduced features tailored to AI content analysis, focusing on keyword optimization, content relevance, and SERP features specific to AI-generated content. These tools provide SEO professionals with data-driven insights into how AI content performs against human-generated content, enabling more targeted optimizations.
Furthermore, AI-specific plugins for WordPress, such as Yoast SEO’s AI content analysis tool, help in fine-tuning the SEO aspects of content generated by LLMs. These tools assess readability, keyword density, and structural SEO elements, adapting the traditional SEO checklist to the nuances of AI-generated text.
Emerging Technologies in LLM SEO
Emerging technologies in LLM SEO include advanced natural language processing (NLP) tools and machine learning algorithms that can predict how LLMs like GPT-3 interpret and prioritize content. Tools such as Clearscope and MarketMuse use AI to provide content strategies that are not only optimized for human readers but also structured in a way that resonates with LLMs, enhancing the content’s visibility and engagement.
Another significant advancement is the development of AI-driven semantic analysis tools that can interpret the context and subtlety of language used in AI-generated content, providing deeper insights into content optimization strategies that work best with LLMs.
The introduction of LLMs.txt
tldr; Inspired by robots.txt, llms.txt (see llmstxt.org, directory.llmstxt.cloud, llmstxt.site) offers website owners control over how LLMs access their content. Placed at a domain’s root, this file signals preferences for model training or inference, promoting transparency and consent. Though early in adoption, it provides a standardized way to set usage boundaries for AI providers.
—
As AI continues to reshape how content is discovered, indexed, and used, website owners are looking for new ways to control how their material is accessed by large language models (LLMs). Enter llms.txt …a proposed standard inspired by the familiar robots.txt file. While robots.txt has long served as a directive for search engine crawlers, llms.txt is designed specifically for AI companies and LLM providers, offering a way to signal whether and how your content should be used in model training or inference. By placing this plain text file at the root of a domain, publishers can make their preferences visible to AI crawlers in a standardized, accessible format.
The purpose of llms.txt is to promote transparency and consent in the evolving AI ecosystem. As companies like OpenAI, Anthropic, Google, and others build models trained on publicly available web content, website owners are increasingly asserting their rights over how their data is utilized. With llms.txt, site administrators can opt in, opt out, or selectively allow specific LLMs to use their content much like how robots.txt lets sites control search engine access. While still in its early stages of adoption, the presence of an llms.txt file can serve as a clear signal to AI providers that your content comes with usage expectations, licensing terms, or boundaries they are encouraged to respect.
Ethical Considerations and Future Trends in AI SEO
Ensuring Ethical Optimization Practices
As AI integration within SEO deepens, ethical considerations must be at the forefront of any strategy. The deployment of AI, especially in LLM Optimization (LEO), raises significant questions regarding data privacy, user consent, and transparency. SEO professionals must ensure that AI tools are used in a manner that respects user privacy and complies with applicable data protection laws. This includes the ethical sourcing and handling of data used to train AI models, ensuring it is devoid of bias and respects the intellectual property rights of the data sources.
Predictions for the Evolution of AI in SEO
The future of AI in SEO points towards more sophisticated, personalized search experiences, propelled by deeper neural networks and machine learning algorithms. As AI becomes adept at understanding user intent and context, we can anticipate a shift from keyword-centric strategies to more holistic, content-driven approaches. This evolution will likely see AI not just as a tool for optimization, but as an integral part of content creation, capable of generating insights that inform content strategy and user engagement tactics.
Staying Ahead: Continuous Learning and Adaptation
For SEO professionals, staying relevant in a rapidly evolving field means continuous learning and adaptation. As AI technologies advance, the need for SEO experts to understand and leverage these changes becomes crucial. Engaging with ongoing training programs, staying updated with the latest AI developments, and experimenting with new AI tools will be essential. Additionally, contributing to and learning from professional communities will help in navigating the ethical complexities and technological shifts in AI SEO.
As AI continues to reshape the SEO landscape, ethical practices, continual learning, and adaptive strategies will be the pillars supporting successful SEO outcomes.
The integration of AI and LLM (Large Language Models) introduces a new frontier termed LLM Optimization (LEO). This concept extends traditional SEO strategies into the realm of optimizing content not just for human audiences but also for AI-driven algorithms and systems. LEO is particularly significant as search engines and digital assistants increasingly rely on these models to understand and organize the vast amounts of information on the internet.
For SEO professionals, the challenge and opportunity lie in mastering LLM-specific optimization strategies. This involves understanding the nuances of how these models interpret text, context, and user intent. Unlike standard SEO, which primarily focuses on keywords and backlinks, LEO requires a deeper engagement with the quality of information, its contextual relevance, and its ability to offer comprehensive answers to complex queries.
The broader implication for SEO professionals is the shift towards a more dynamic understanding of search engine algorithms, requiring continuous learning and adaptation to stay ahead. As AI becomes a more dominant player in search processes, SEO strategies must evolve to meet the complex demands of both human users and sophisticated algorithms, ensuring visibility and relevance in a highly competitive digital ecosystem.
I hope the above has given you a preview, an outline of sorts and some insight. The potential for a company, business, or website to be cited in AI-generated material represents a significant opportunity to increase visibility and reach new audiences.